Machine learning models are increasingly augmenting human processes, either performing repetitious tasks faster or providing some systematic insight that helps put human knowledge in perspective. Astronomers at UC Berkeley were surprised to find both happen after modeling gravitational microlensing events, leading to a new unified theory for the phenomenon.
Gravitational lensing occurs when light from far-off stars and other stellar objects bends around a nearer one directly between it and the observer, briefly giving a brighter — but distorted — view of the farther one. Depending on how the light bends (and what we know about the distant object), we can also learn a lot about the star, planet, or system that the light is bending around.
For example, a momentary spike in brightness suggests a planetary body transiting the line of sight, and this type of anomaly in the reading, called a “degeneracy” for some reason, has been used to spot thousands of exoplanets.
Due to the limitations of observing them, it’s difficult to quantify these events and objects beyond a handful of basic notions like their mass. And degeneracies are generally considered to fall under two possibilities: that the distant light passed closer to either the star or the planet in a given system. Ambiguities are often reconciled with other observed data, such as that we know by other means that the planet is too small to cause the scale of distortion seen.
UC Berkeley doctoral student Keming Zhang was looking into a way to quickly analyze and categorize such lensing events, as they appear in great number as we survey the sky more regularly and in greater detail. He and his colleagues trained a machine learning model on data from known gravity microlensing events with known causes and configurations, then set it free on a bunch of others less well quantified.
The results were unexpected: in addition to deftly calculating when an observed event fell under one of the two main degeneracy types, it found many that didn’t.
“The two previous theories of degeneracy deal with cases where the background star appears to pass close to the foreground star or the foreground planet. The AI algorithm showed us hundreds of examples from not only these two cases, but also situations where the star doesn’t pass close to either the star or planet and cannot be explained by either previous theory,” said Zhang in a Berkeley news release.
Now, this could very well have resulted from a badly tuned model or one that simply wasn’t confident enough in its own calculations. But Zhang seemed convinced that the AI had clocked something that human observers had systematically overlooked.
As a result — and after some convincing, since a grad student questioning established doctrine is tolerated but perhaps not encouraged — they ended up proposing a new, “unified” theory of how degeneracy in these observations can be explained, of which the two known theories were simply the most common cases.
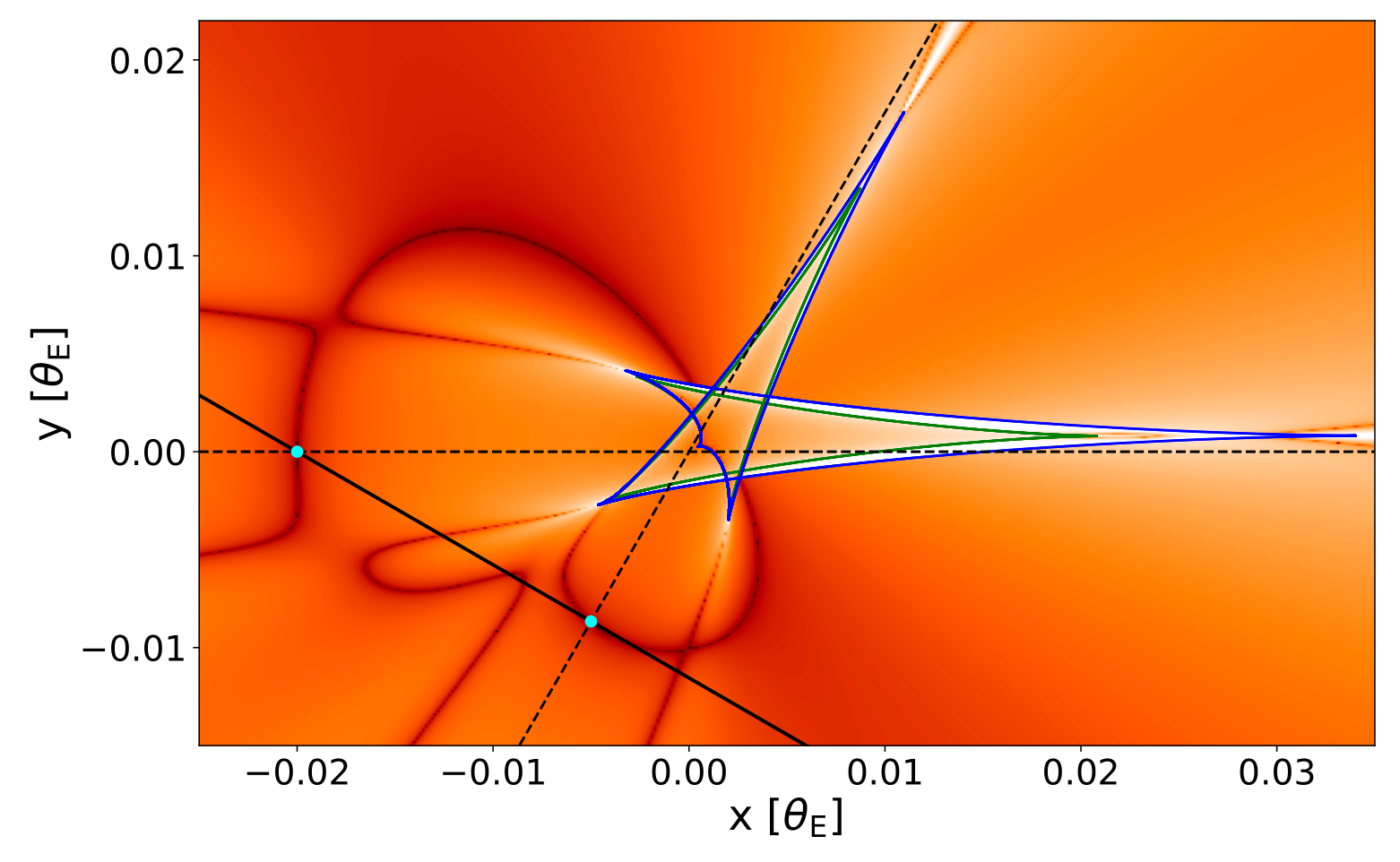
Diagram showing a simulation of a 3-lens degeneracy solution.
They looked at two dozen recent papers observing microlensing events and found that astronomers had been mistakenly categorizing what they saw as one type or the other when the new theory fit the data better than both.
“People were seeing these microlensing events, which actually were exhibiting this new degeneracy but just didn’t realize it. It was really just the machine learning looking at thousands of events where it became impossible to miss,” said Scott Gaudi, an Ohio State University astronomy professor who co-authored the paper.
To be clear, the AI didn’t formulate and propose the new theory — that was entirely down to the human intellects. But without the systematic and confident calculations of the AI, it’s likely the simplified, less correct theory would have persisted for many more years. Just as people learned to trust calculators and later computers, we are learning to trust some AI models to output an interesting truth clear of preconceptions and assumptions — that is, if we haven’t just coded our own preconceptions and assumptions into them.
The new theory and description of the process leading up to it are described in a paper published in the journal Nature Astronomy. It’s probably not news to the astronomers among our readership (it was a pre-print last year) but the machine learning and general science wonks may cherish this interesting development.